Depression is a prevalent yet often misunderstood condition. According to most estimates, it affects over 5% of the world’s population. Depression is characterized by persistent feelings of sadness, loss of interest, and changes in mood and behavior; its impact is personal, but these impacts cascade into families and even society as a whole. Oftentimes, depression is also misunderstood and undiagnosed — that’s why diagnosing depression early is so important.
With this in mind, researchers from Dartmouth have developed the first smartphone app that merges AI and facial-image processing software to reliably detect the onset of depression. According to preliminary results, this can work even before the user knows something is wrong.
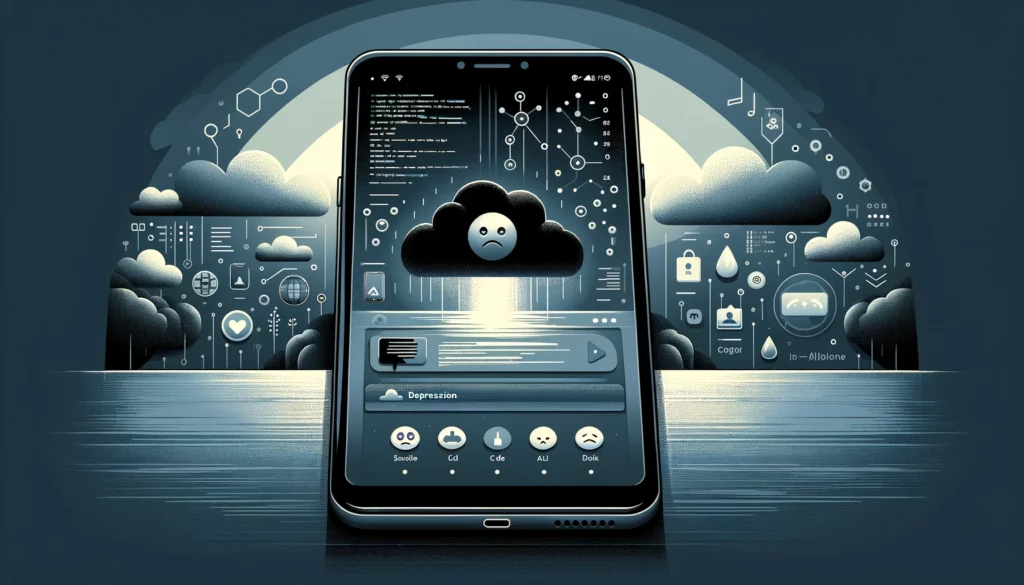
Depression is more than just feeling blue. It’s a serious medical condition typically characterized by dark emotions and a plethora of both emotional and physical problems. Clinical depression can last for months or even indefinitely, significantly impairing individual’s ability to function and enjoy life.
Researchers at Dartmouth thought there could be some signs of this in people’s facial expressions. Given the recent advent of facial processing software and Artificial Intelligence (AI), it seemed like a good bet.
So they embarked on a study with 177 people diagnosed with major depressive disorder.
Signs of depression
The app, called MoodCapture, builds on previous research that started in 2015. It uses a phone’s front camera to take photos of people’s facial expressions. This happens as people regularly use their phone, there’s no special use required. In fact, participants didn’t even know when the app was taking pictures (something which they consented to).
“Our shift towards the MoodCapture project was inspired by the initial insights gained from exploring the use of everyday smartphone interactions for mental health assessment,” study co-author Subigya Nepal, told ZME Science.
At first, the researchers observed the genuine emotions captured by smartphones during routine unlocks. This sparked the idea of analyzing these expressions to assess mental health states. For now, MoodCapture doesn’t analyze images directly on the device, but it can transfer them for external analysis.
Overall, the app captured 125,000 images of participants over the course of 90 days. After training, the app correctly identified symptoms of depression with 75% accuracy.
“This is the first time that natural ‘in-the-wild’ images have been used to predict depression,” said Andrew Campbell, the paper’s corresponding author and Dartmouth’s Albert Bradley 1915 Third Century Professor of Computer Science. “There’s been a movement for digital mental-health technology to ultimately come up with a tool that can predict mood in people diagnosed with major depression in a reliable and non-intrusive way.”
The app looks at things like gaze, eye movement, and head position to look for signs of depression.
“We identify depression signs in the following way. First, we extract features that quantify specific features in the face. For example, some landmark features indicate the coordinates of the edges of the user’s lip. Next, we use these features to train a machine learning (Random Forest) model to predict depression. Finally, we observed that features on the right side of the face are more indicative of depressive symptoms. This asymmetry is related to the way the user holds and interacts with the phone,” Nepal added in an email.
But it doesn’t only use facial analysis to make its conclusion. It correlates the facial analysis cues with self-reports of feeling depressed or down, as well as environmental factors from the photos (things like color, lighting, and the number of people in the image).
The doctor in your pocket
This research is part of a growing movement in medical science focusing on smartphones or other devices we interact with daily for early diagnosis. Even though it’s not a medical-grade device, a smartphone comes with a different advantage: we use it a lot.
“People use facial recognition software to unlock their phones hundreds of times a day,” said Campbell, whose phone recently showed he had done so more than 800 times in one week.
“MoodCapture uses a similar technology pipeline of facial recognition technology with deep learning and AI hardware, so there is terrific potential to scale up this technology without any additional input or burden on the user,” he said. “A person just unlocks their phone and MoodCapture knows their depression dynamics and can suggest they seek help.”
Nicholas Jacobson, a study co-author also at Dartmouth, says this brings another advantage, specifically for depression diagnosis. Depression symptoms come and go, and doctors may miss the best timing for diagnosis. Meanwhile, our smartphones are always with us.
“Many of our therapeutic interventions for depression are centered around longer stretches of time, but these folks experience ebbs and flows in their condition. Traditional assessments miss most of what depression is,” said Jacobson, who directs the AI and Mental Health: Innovation in Technology Guided Healthcare (AIM HIGH) Laboratory.
“Our goal is to capture the changes in symptoms that people with depression experience in their daily lives,” Jacobson said. “If we can use this to predict and understand the rapid changes in depression symptoms, we can ultimately head them off and treat them. The more in the moment we can be, the less profound the impact of depression will be.”
Still a few years from clinical practice
The new study works as a proof of concept, showing remarkable promise. Misdiagnosis rates for depression are huge, reaching up to 66% according to some studies. Having even an imperfect app could be a godsend for early depression diagnosis. Furthermore, the app can be tweaked with users’ own personal data to improve performance even more.
“Currently, MoodCapture is general model that does not adapt to a specific user. However, we are working on a follow-up study that personalizes MoodCapture to the specific user. Our preliminary results suggest a ~15% improvement over current performance and potential to improve fairness and privacy, which addresses many of our participants concerns. More information on this coming out soon,” sasy Nepal.
Researchers estimate that it may still take up to 5 years before the technology will hit the market.
However, Nepal says that there’s already good progress. The science is there — it’s just about making the most out of the data.
“You wouldn’t need to start from scratch — we know the general model is 75% accurate, so a specific person’s data could be used to fine-tune the model. Devices within the next few years should easily be able to handle this,” Nepal said. “We know that facial expressions are indicative of emotional state. Our study is a proof of concept that when it comes to using technology to evaluate mental health, they’re one of the most important signals we can get.”
For now, researchers are working on improving MoodCapture in three ways: personalization, fairness, and privacy.
“In our next steps, we are extending MoodCapture models to incorporate personalized sm models akin to face recognition on smartphones. Our preliminary results suggest a ~15% improvement over current performance. Using personalized models allows us to focus on individual fairness, i.e., we seek to develop models that have minimal variance in performance across our users. To improve privacy, we will explore federated learning where the users face images do not leave their mobile devices,” Nepal concludes.
The team published their paper on the arXiv preprint database in advance of presenting it at the Association of Computing Machinery’s CHI 2024 conference in May