The Charlotte-Mecklenburg Police Department of North Carolina is piloting a new machine-learning system which it hopes will combat the rise of police violence. Police brutality has been a growing issue in US in recent years.
The system combs through the police’s staff records to identify officers with a high risk of causing “adverse events” — such as racial profiling or unwarranted shootings.

A University of Chicago team is helping the Charlotte-Mecklenburg PD keep an eye on their police officers, and prevent cases of police violence. The team feeds data from the police’s staff records into a machine learning system that tries to spot risk factors for unprofessional conduct. Once a high-risk individual is identified, the department steps in to prevent any actual harm at the hands of the officer.
Officers are people too, and they can be subjected to a lot of stress in their line of work. The system is meant to single out officers who might behave aggressively under stress. All the information on an individual’s record — details of previous misconduct, gun use, their deployment history, how many suicide or domestic violence calls they have responded to, et cetera — is fed into the system. The idea is to prevent incidents in which officers who are stressed can behave aggressively, such as the case in Texas where an officer pulled his gun on children at a pool party after responding to two suicide calls earlier that shift.
“Right now the systems that claim to do this end up flagging the majority of officers,” says Rayid Ghani, who leads the Chicago team. “You can’t really intervene then.”
But so far, the system had some pretty impressive results. It retrospectively flagged 48 out of 83 adverse incidents that happened between 2005 and now – 12 per cent more than Charlotte-Mecklenberg’s existing early intervention system. It had a false positive rate – officers flagged as having a high risk by the system that didn’t behave aggressively – was 32 per cent lower than the existing systems.
Ghani’s team is currently testing the system with the Los Angeles County Sheriff’s Department and the Knoxville Police Department in Tennessee. They will present the results of their pilot system at the International Conference on Knowledge Discovery and Data Mining in San Francisco later this month.
So the system works, but exactly what should be done after an official has been flagged as a potential risk is still up for debate. The team is still working with the Charlotte-Mecklenburg police to find the best solution.
“The most appropriate intervention to prevent misconduct by an officer could be a training course, a discussion with a manager or changing their beat for a week,” Ghani adds.
Whatever the best course of action is, Ghani is confident that it should be implemented by humans, not a computer system.
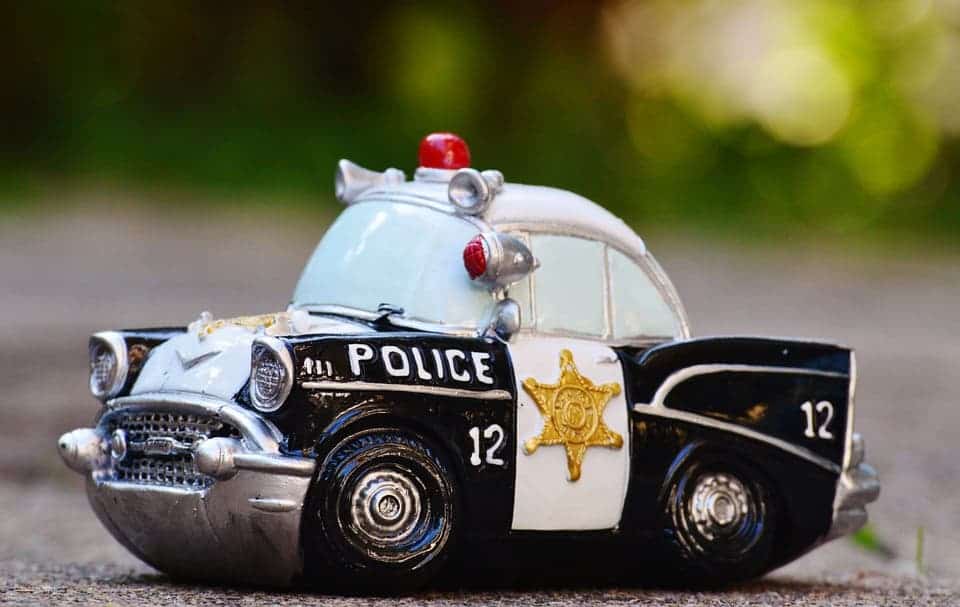
Image via pixabay
“I would not want any of those to be automated,” he says. “As long as there is a human in the middle starting a conversation with them, we’re reducing the chance for things to go wrong.”
Frank Pasquale, who studies the social impact of algorithms at the University of Maryland, is cautiously optimistic.
“In many walks of life I think this algorithmic ranking of workers has gone too far – it troubles me,” he says. “But in the context of the police, I think it could work.”
He believes that while such a system for tackling police misconduct is new, it’s likely that older systems created the problem in the first place.
“The people behind this are going to say it’s all new,” he says. “But it could be seen as an effort to correct an earlier algorithmic failure. A lot of people say that the reason you have so much contact between minorities and police is because the CompStat system was rewarding officers who got the most arrests.”
CompStat, short for Computer Statistics, is a police management and accountability system, used to implement the “broken windows” theory of policing — the idea that punishing minor infractions like public drinking and vandalism severely helps create an atmosphere of law and order, and will thus bring down serious crime. Many police researchers have suggested that the approach has led to the current dangerous tension between police and minority communities.
Pasquale warns that the University of Chicago system is not infallible. Just like any other system, it’s going to suffer from biased data — for example, a black police officer in a white community will likely get more complaints than a white colleague, he says, because the police can be subject to racism, too. Giving officers some channel to seek redress will be important.
“This can’t just be an automatic number cruncher.”