People can learn to predict what a speaker will say after a disfluency (such as ‘um’ or ‘aaah’). However, this only seems to work with those that share their native tongue, not foreigners.
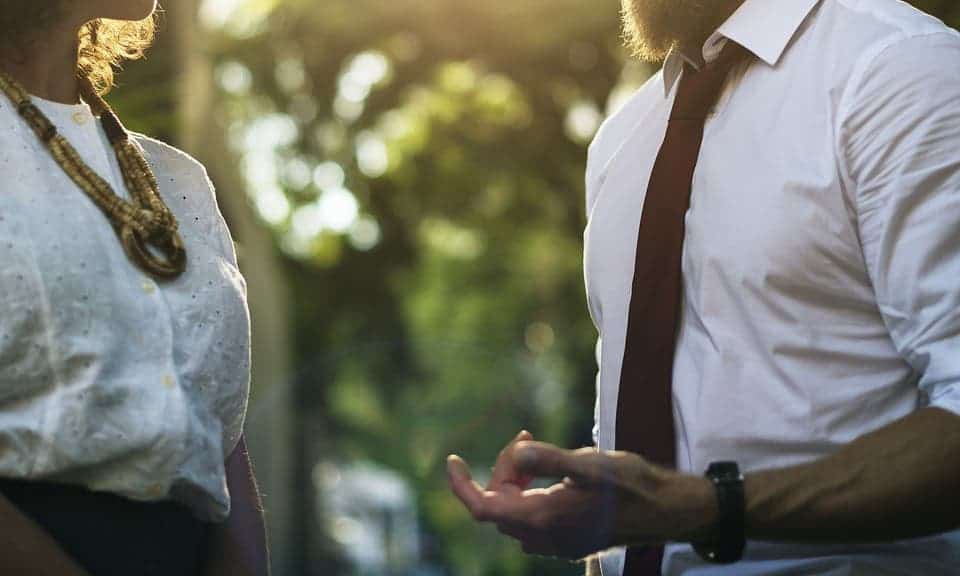
Even flowing conversation is peppered with disfluencies — short pauses and ‘umm‘s, ‘ahh’s, ‘ugh’s. On average, people produce roughly 6 disfluencies per 100 words. A new paper reports that such disfluencies do not occur randomly — they typically come before ‘hard-to-name’ or low-frequency words (such as ‘automobile’ instead of ‘car’).
The team notes that, while previous research has shown that people can use disfluencies to predict when such a low-frequency (uncommon) word is incoming, no research has established whether listeners would actively track the occurrence of ‘uh’, even when it appeared in unexpected places. And that’s exactly what this present study wanted to find out.
Small pauses for big words
The team asked two groups of Dutch participants (41 in total, 30 of which produced useable data) to look at sets of two images on a screen (one ‘common, such as a hand, and an ‘uncommon’ one such as an igloo) while listening to both fluent and disfluent instructions. These instructions would tell participants to click on one of the two images. One of the groups received instructions spoken in a ‘typical’ manner — in which the talker would say ‘uh’ before low-frequency words — while the other group received ‘atypical’ instructions — in which the talker said ‘uh’ before high-frequency words.
Eye-tracking devices were used to keep track of where each participant was looking during the trial. What the team was interested in finding was whether participants in the second group would keep track of the unexpected ‘uh’s and would learn to expect the common object after them.
At the start of the experiment, participants listening to ‘typical’ instructions immediately looked at the igloo upon hearing the disfluency, as did those in the atypical group. Note that the team intentionally left a relatively long pause between the ‘uh’ and the following word, so the participants looked at an object even before hearing the word itself. However, people in the atypical group quickly learned to adjust this natural prediction and started looking at the common object upon hearing a disfluency.
“We take this as evidence that listeners actively keep track of when and where talkers say ‘uh’ in spoken communication, adjusting what they predict will come next for different talkers,” explains lead author Hans Rutger Bosker from the Max Planck Institute for Psycholinguistics.
The team also wanted to see if this effect would hold for non-native speakers. In a follow-up experiment — one that used the same set-up and instructions but this time spoken with a heavy Romanian accent — participants learned to predict uncommon words following the disfluencies of a ‘typical’ (‘uh’ before low-frequency words) non-native talker. However, they didn’t start predicting high-frequency words in an ‘atypical’ non-native speaker, despite the fact that the same sentences were used in the native and non-native experiments.
“This probably indicates that hearing a few atypical disfluent instructions (e.g., the non-native talker saying ‘uh’ before common words like “hand” and “car”) led listeners to infer that the non-native speaker had difficulty naming even simple words in Dutch,” says co-author Geertje van Bergen.
“As such, they presumably took the non-native disfluencies to not be predictive of the word to follow — in spite of the clear distributional cues indicating otherwise.”
The findings suggest an interplay between ‘disfluency tracking’ and ‘pragmatic inferencing’, according to the team. In non-science speak, that largely means we only track disfluencies if the talker’s voice makes us believe they are a reliable umm’er.
“We’ve known about disfluencies triggering prediction for more than 10 years now, but we demonstrate that these predictive strategies are malleable. People actively track when particular talkers say ‘uh’ on a moment by moment basis, adjusting their predictions about what will come next,” explains Bosker.
The paper “How tracking the distribution of native and non-native disfluencies influences online language comprehension” has been published in the Journal of Memory and Language.