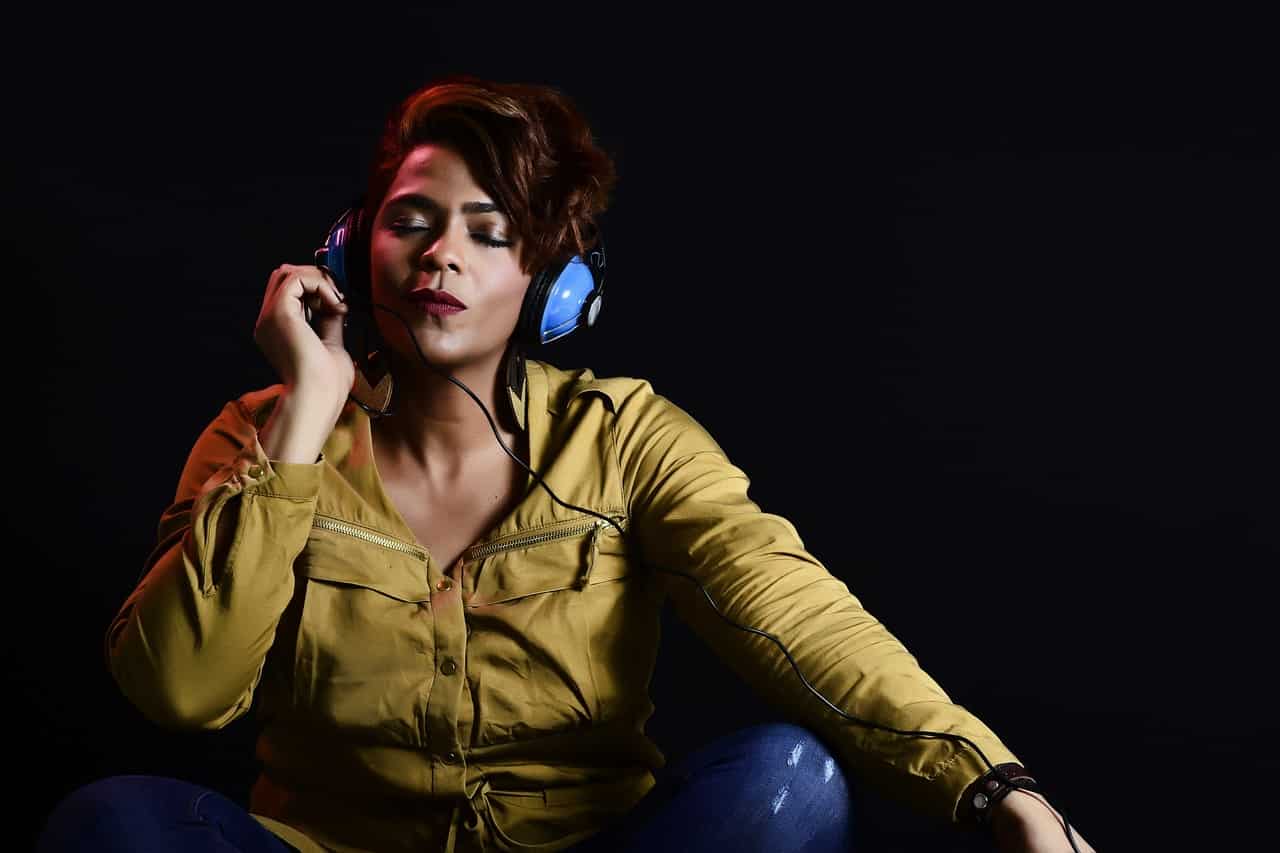
Every day, we are flooded with a deluge of new music. Tens of thousands of new songs are uploaded daily to streaming platforms like Spotify and Pandora. Yet relatively few songs are played more than a dozen times (not counting the artist’s friends and family). Amid this torrent of musical choices, streaming services and radio stations face the Herculean task of picking which songs deserve a spot on their playlists.
Until now, they’ve relied on a blend of human intuition and computer algorithms, achieving a hit prediction accuracy rate that barely beats a coin toss. But a game-changing approach, where machine learning meets neuroscience, promises to change the tune of song selection. In a new study, researchers reported an astounding 97% accuracy in hit prediction.
Can brain signals predict the next hit song?
Researchers from the US have delved deep into how our brains respond to music by applying machine-learning techniques to neurophysiological data. The most awesome thing about it all? They never had to directly measure a person’s actual neural response.
“My lab previously identified what appears to be the brain’s valuation system for social and emotional experiences which I have called Immersion. In talks with a streaming service, they told me that they struggle to suggest new music for subscribers due to the high volume of new music. I thought measuring neurologic Immersion could help solve this problem,” Paul J. Zak, a professor at Claremont Graduate University and senior author of the new study, told ZME Science.
Zak received 24 recently released songs from a streaming service, along with three months of data after the release. This included the number of plays, additions to users’ playlists, and other useful metrics.
The set of songs included both “hits” and “flops”, where a song is considered a hit as long as it has raked in at least 700,000 streaming listens in the last six months. The hit songs spanned genres that included rock (Girl In Red “Bad Idea”), hip-hop (Roddy Rich “The Box”), and EDM (Tons and I “Dance Monkey”).
The researchers recruited 33 participants from the Claremont campus and the surrounding community, who were fitted with non-invasive neurophysiological recording devices. These are commercially available, off-the-shelf devices such as cardiac sensors on smartwatches.
This data was then fed into the Immersion Neuroscience platform, which Zak founded. The platform uses this data, such as a person’s heart rate, to infer neural states from the activity of cranial nerves.
The researchers compiled this data into a single measure they call “immersion”, a state of deep engagement or absorption experienced by individuals while listening to music. These second-hand brain signals are supposed to reflect activity in brain networks linked to mood and energy levels.
For instance, when the brain is subjected to emotional stimuli, such as music, dopamine is released and binds to the prefrontal cortex while oxytocin is released from the brainstem. These neurotransmitters and hormones have downstream effects on the human body that eventually translate into measurable physiological responses, such as your heart rate.
It’s a more limited approach than, say, directly imaging a person’s brain, but the technique can be a viable proxy for determining a person’s neural response to music. Its strength lies in the fact that these signals can be measured with something as simple as a smartwatch or fitness armband, whereas brain activity is typically recorded using cumbersome lab equipment.
Can you trust your own ears?
In addition to the neurophysiological data, the researchers also surveyed subjective evaluations of the songs. The participants were asked how much they liked the song, if they thought they would replay it again in the future, whether they’d be inclined to recommend it to a friend, or whether they had heard it before.
This small survey suggests that the songs that people said they liked were statistically related to the number of streams — but only when the participants knew the songs beforehand. For unfamiliar songs, when participants identified a song that they liked, their evaluation was statistically identical for hits and flops.
In other words, for songs that we don’t know, we seem unable to accurately predict whether a song will grow into a banger or not. But our body’s response to music may be more attuned to the qualities of popular music.
Following data collection, the team deployed a variety of statistical techniques to gauge the predictive prowess of their neurophysiological variables. It was a race, with different models vying for the highest prediction outcomes. To further refine their predictions, they trained a machine learning model, exploring different algorithms until they achieved the highest possible accuracy.
The results were remarkable. A linear statistical model successfully identified hit songs with a rate of 69%. However, when machine learning was applied to the collected data, the accuracy skyrocketed to an impressive 97%.
“Why? Machine learning captures the inherent nonlinearity of the brain and thus better measures how much the brain values an experience like listening to new music,” Zak said.
The team also delved deeper, applying machine learning to the neural responses to the first minute of songs, achieving an 82% success rate in identifying hits.
Termed “neuroforecasting,” this methodology leverages the neural activity of a small group of individuals to predict the responses of a larger population. Previously, neuroforecasting has been used to predict stock market swings, which video will go viral, and who wins an election.
“This approach is gaining momentum in neuroscience and is called “brain as predictor” or “neuroforecasting”, i.e. using neural activity from a moderate number of people to predict overall behavior by hundreds of thousands of people,” Zak said.
“Over the last 20 years, my research meticulously mapped out the relationship between the brain and the peripheral nervous system so we can, in real-time and without expensive technology, capture what the brain values second by second. While the data collection using the Immersion commercial platform can be done by anyone in real-time, building a predictive machine learning model took some effort by myself and my very smart grad students.”
The future of the music business
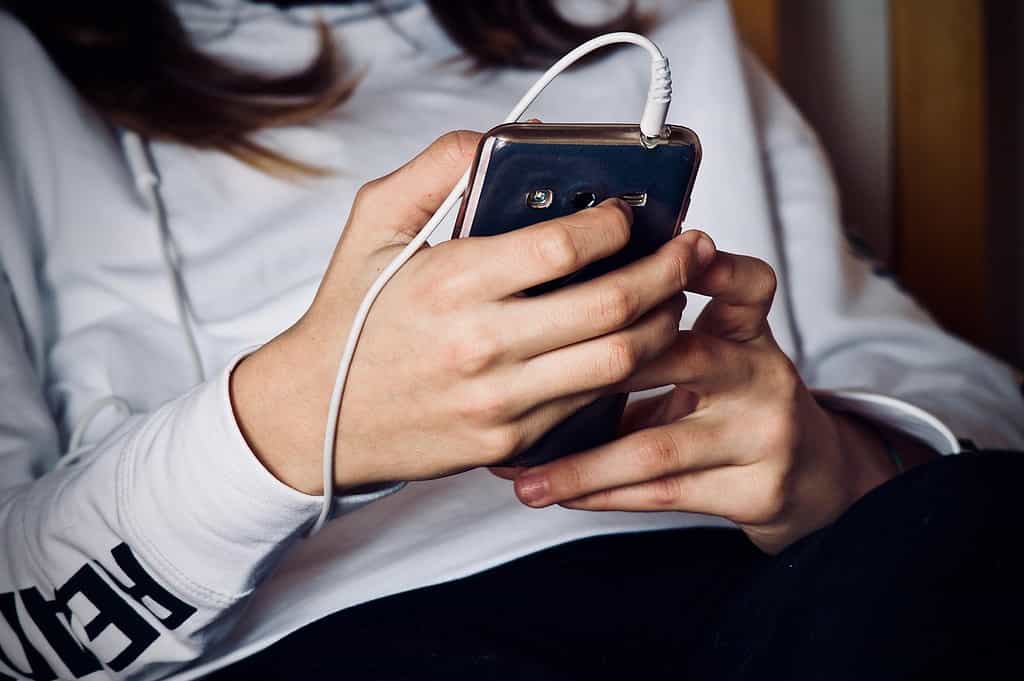
Does all of this mean that these algorithms can be used to predict the next hit song with 97% accuracy? That’d be something but to quote Zak, “not exactly”. The sample size for both the participants and the songs themselves is rather tiny. People’s tastes in music can vary greatly and they are often highly influenced by culture.
Nevertheless, the very large effect size suggests that this approach could very well predict hits and flops over a wider range of songs and genres. Most certainly, this would get the attention of both streaming services and creators. Ultimately, this could impact you as well — the listener.
“Since no special hardware is needed and fitness wearables and smartwatches are quite common, streaming services could send subscribers music based on their current moods/neurologic states and have a high likelihood that people would enjoy the music rather than ask them to consciously pick new music. People with wearables could also be incentivized to listen to new music, even a snippet of it as we show in the paper, to help streaming services choose which music to promote,” Zak said.
In a future where neuroscience technology becomes as common as smartphones, we might see our entertainment choices tailored based on our neurophysiology. It’s like having a personal assistant who listens to your body, handpicking a few perfect options from the whirlwind of possibilities, making your music selection process faster and more enjoyable.
Moreover, there’s no reason why the same approach can’t be used to tailor user selection for other emotionally impactful media, such as movies or TV shows.
“I hate to see wasted effort. A real value of our approach is to help young artists gain the intuition for how to create hits that older artists/bands have gotten through trial and error. For example, a young artist could create a new song, measure neurologic Immersion, and then modify the song to increase Immersion and its likelihood of being a hit,” Zak said.
“This solves a philosophical dilemma all artists face: They presume if they love the content they make the other people will. By measuring Immersion they can quickly learn what people will love. This can help stimulate their creativity. It also makes consumers of content happier. Win-win!”
The findings were reported in the journal Frontiers in Artificial Intelligence.