Imagine donning a virtual reality headset and stepping into the past, immersing yourself in a bygone neighborhood. With every stride, you witness the streets and buildings as they stood decades ago.
This tantalizing vision has become a reality through an innovative method that utilizes machine learning and maps to create 3D digital models of old neighborhoods — even those that have been torn down to make way for new real estate development.
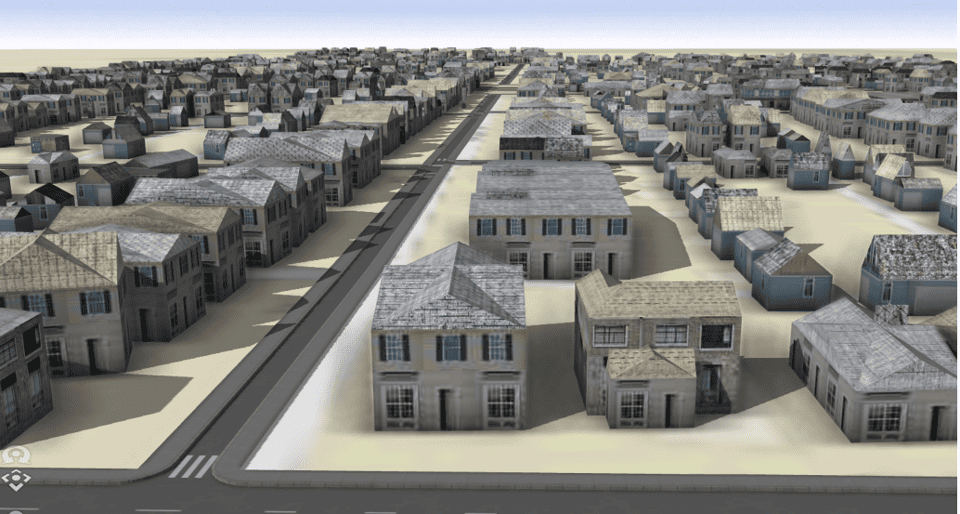
These digital models can serve as the backbone for certain studies that were previously very challenging to do. For instance, this technology now makes it easier to estimate the economic impact of demolishing historic neighborhoods. With access to these 3D models, researchers will be able to do such analyses with more accuracy and insight.
The foundation of this research lies in the Sanborn maps, originally designed to assist fire insurance companies in assessing risk exposure across thousands of cities and towns in the US during the 19th and 20th centuries.
“I worked with these maps when I was a graduate student back in the 1980s, but extracting data from the maps was a very time consuming and tedious manual process. After seeing the maps were available in digital form, we thought that we could develop a semi-automated way to extract the data from these maps,” Harvey Miller, co-author of the study and professor of geography at The Ohio State University, told ZME Science.
Rebuilding the past

Miller and his team devised machine learning tools capable of extracting information from the Sanborn maps. This includes specific details about individual buildings, such as their precise locations, footprints, number of floors, construction materials, and main purpose, such as residential or commercial.
They tested their new methodology on two neighboring areas situated on the near east side of Columbus, Ohio that were largely destroyed during the 1960s to facilitate the construction of an urban highway. One of the neighborhoods, Hanford Village, for example, was developed in 1946 to house returning Black veterans of World War II.
“By visualizing the neighborhoods before highway construction, we want to have a visceral sense of what was lost to urban highways and urban renewal” Miller added.
“We also want to make measurements of the capital and wealth-generating activities that were damaged, and the environmental justice issues that were generated.”
The team focused on 13 Sanborn maps generated in 1961, right before the construction of the highway. They used machine learning techniques to extract data from these maps and generate digital models of neighborhoods. They discovered that almost 400 structures such as houses and stores were destroyed to make way for the highway.
The machine learning model proved very accurate in recreating the information contained in the maps – about 90% accurate for building footprints and construction materials. The researchers now believe they could create 3D models for almost all the 12,000 cities and towns that are covered by the Sandborn maps.
“Our methods will allow the creation of detailed, 3D models of entire neighborhoods and cities as they existed in history. This can enable tracking of changes in urban land use, the development and evolution of urban morphology and new insights into the shifting patterns of urban development, demolition, and redevelopment,” Miller told ZME Science
The study was published in the journal PLOS ONE.