Cuneiform is one of the earliest writing systems in human history. Archaeologists have traced it back to 3400 BC, a whopping 5,400 years ago. It also lasted for a pretty long time, over 3,000 years. Researchers have found thousands of texts written in cuneiform in the Sumerian and Akkadian languages — now, they’ve trained a neural network that can translate these texts into English effortlessly.
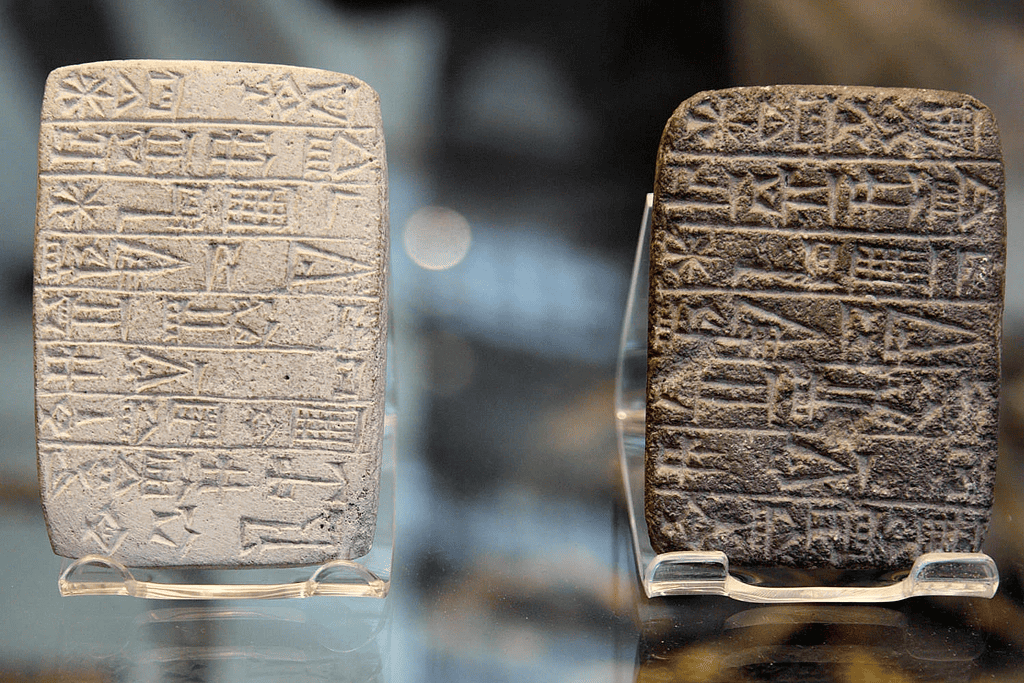
An old, mysterious language
The Akkadian language is one of the earliest known Semitic languages, a family that includes modern languages such as Arabic and Hebrew. It was spoken in ancient Mesopotamia, primarily in the Akkadian Empire that was situated in the region that is today parts of Iraq and northeastern Syria. Akkadian is named after the ancient city of Akkad, one of the major centers of the Akkadian civilization.
Akkadian was used for a wide range of purposes, from administrative and legal documents to literature and science texts. It was written using cuneiform script on clay tablets, and its decipherment in the 19th century opened up a new window into the ancient world, providing scholars with valuable insights into the history, culture, and scientific achievements of the time.
Meanwhile, Sumerian is one of the world’s oldest known languages, and it has the distinction of being a language isolate, meaning it has no known relatives. It was spoken in ancient Sumer, a region located in the southern part of what is now modern-day Iraq. The Sumerians are credited with establishing one of the world’s earliest civilizations around 4500 BCE, and their society flourished until about 2000 BCE.
Both of these languages used the cuneiform writing system, as did several other languages. But translating cuneiform has proven to be very challenging.
The full decipherment of cuneiform took over 200 years, from 1802 to 2022. The story starts with the so-called Behistun Inscription. Discovered in Iran and dating back to the time of King Darius I of Persia (550 BC), this multilingual inscription included three types of script: Old Persian, Elamite, and Akkadian cuneiform. Old Persian was deciphered first, providing clues for the other two.
Scholars gradually worked on deciphering and understanding cuneiform, and after a few Eureka moments and a lot of hard work, they finally achieved a good understanding of the cuneiform. But for some researchers, this wasn’t enough. They wanted to make translating cuneiform more available — so they turned to artificial intelligence (AI).
Cuneiform, meet AI
In recent years, language translations have come a long way — and AI is greatly accelerating these trends in automation. AI translations are nearing a watershed moment, with some pretty striking achievements. In the new study, Shai Gordin and colleagues from Ariel University described an AI model that can automatically translate Akkadian text written in cuneiform into English. For now, this is only available for this particular language (not all languages that use the cuneiform script work at the moment), but it’s still remarkable.
This is a follow-up to a previous study by Gordin and colleagues that also looked at how AI can be used to translate cuneiform. This time, two versions of the model were trained. The first one translates the Akkadian from cuneiform representations into Lain script (called transliteration). The other version translates from unicode representations of cuneiform signs (which is how cuneiform is often digitized).
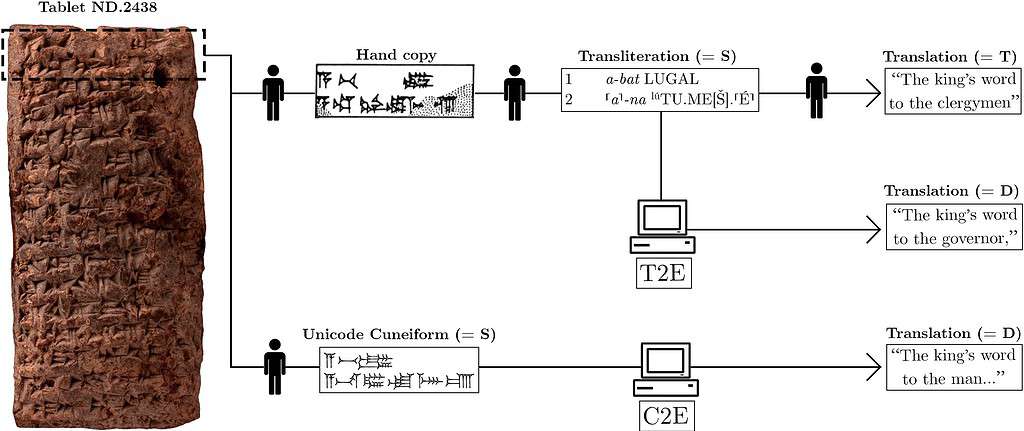
The first version gave better results in the study, achieving a score of 37.47 in the Best Bilingual Evaluation Understudy 4 (BLEU4).
The Bilingual Evaluation Understudy (BLEU) score is a metric used to evaluate the quality of machine-generated translations. It measures how closely a machine translation of a text matches a set of human-created reference translations. The score ranges from 0 to 1 (or 0 to 100), with higher scores indicating better translations. Even experienced human translators don’t usually get 100, and for a language such as cuneiform, 37 is good enough to get a decent translation.
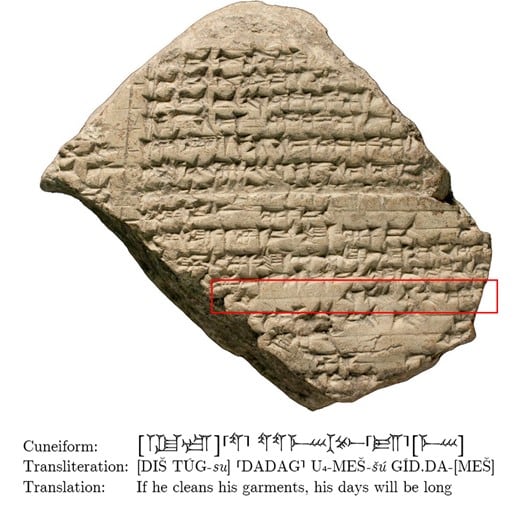
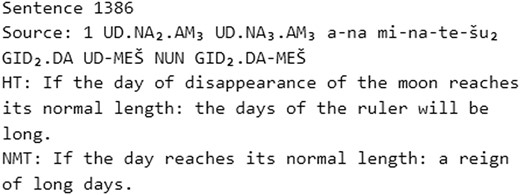
The model achieves the best results in short and medium-length sentences. As the sentences get longer, the model struggles to grasp the entire context — although this can be trained in the future, researchers say. Another shortcoming is that the model also “hallucinates” — it creates outcomes that are syntactically correct but completely decoupled from the meaning of the original text. This is something that other engines, notably ChatGPT, also do sometimes.
Consider the following example:
Sentence 2,753
Source: UD 21-KAM2 LUGAL ina E2-DINGIR E2-DINGIR la ur-rad
Human translation: “On the 21st day the king does not go down to the House of God.”
Machine translation: “On the 21st day the king goes down to the House of God.”
In this case, the AI did a great job of translating most of the content. However, an error that likely occurred when cleaning the data for training caused the AI to miss the negation, completely altering the meaning of the sentence.
In the majority of cases, however, the translation was very useful as a first-pass of the text. Researchers say the AI can be used by scholars or even by students who want to study this language in more detail.
Moreover, as this technology becomes more widespread, it’s not far-fetched to imagine its application in classrooms, museums, and even interactive historical experiences, allowing us to engage with the past in unprecedented ways. It’s a tantalizing glimpse of the potential that lies at the intersection of history and technology, a synthesis that could redefine our understanding of who we are and where we come from.
The study was published in PNAS Nexus.