
Russian researchers have used a non-invasive technique that visualizes the brain activity of a person, recreating surprisingly accurate moving images of what our eyes actually see. The method could someday be employed in cognitive disorder treatment or post-stroke rehabilitation devices that are controlled by a patient’s thoughts.
This is not the first time that scientists have decoded people’s brain activity patterns to generate images. Such methods, however, typically rely on functional MRI or surgically implanted neurons, which can be invasive and cumbersome, thereby limiting the potential for everyday applications.
The new technique developed by researchers at the Moscow Institute of Physics and Technology and Russian corporation Neurobotics is much more versatile. It relies on electroencephalography, or EEG, which records brain waves via electrodes that are placed noninvasively on the scalp.
“The electroencephalogram is a collection of brain signals recorded from scalp. Researchers used to think that studying brain processes via EEG is like figuring out the internal structure of a steam engine by analyzing the smoke left behind by a steam train,” explained paper co-author Grigory Rashkov, a junior researcher at MIPT and a programmer at Neurobotics. “We did not expect that it contains sufficient information to even partially reconstruct an image observed by a person. Yet it turned out to be quite possible.”
During one experiment, healthy volunteers had to watch 20 minutes of 10-second YouTube video fragments. The videos were grouped into five categories: abstract shapes, waterfalls, human faces, moving mechanisms, and motorsports.
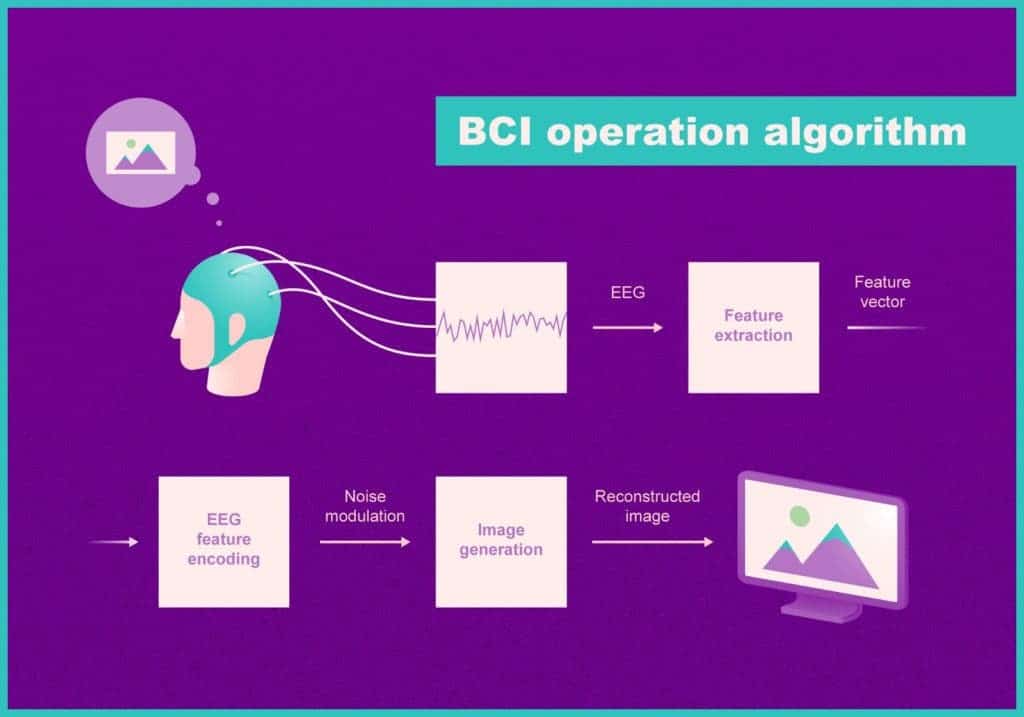
In the first phase of the experiment, the researchers showed that by analyzing the EEG data, they could distinguish between each video category.
For the second part, the researchers developed two neural networks (AI algorithms) and selected three random categories from the original five. One network was responsible for generating random category-specific images from “noise”, whereas the other generated similar “noise” from the EEG data. The two networks then operated together to convert the EEG signal, literally the brain activity, into actual images that mimic what the test subjects were actually observing.
Finally, after the neural networks were trained, the test subjects were shown completely novel videos that they had never seen from the same categories. As they watched the videos, the recorded brain activity was fed into the neural networks. The generated images could be easily categorized with 90% accuracy.
“What’s more, we can use this as the basis for a brain-computer interface operating in real time. It’s fairly reassuring. Under present-day technology, the invasive neural interfaces envisioned by Elon Musk face the challenges of complex surgery and rapid deterioration due to natural processes — they oxidize and fail within several months. We hope we can eventually design more affordable neural interfaces that do not require implantation,” Rashkov said.
The findings were reported in the preprint server bioRxiv.