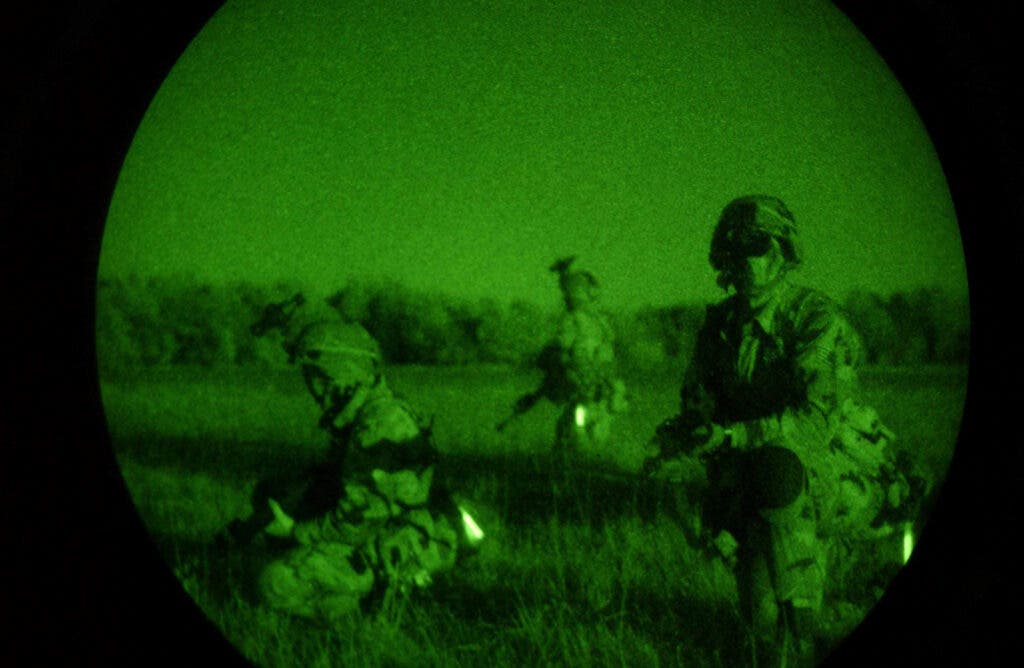
Night vision cameras and goggles have proven to be a game-changer ever since they were invented in the mid-20th century, allowing us to distinguish features and movements even in total pitch darkness. The technology is now crucial to modern military and law enforcement forces, but night vision has proven its worth for scientific and conservation efforts too, such as the surveillance of endangered nocturnal animals.
But, although night vision is super useful, it’s not perfect. Such cameras only produce black-and-white images, which in most cases is an acceptable compromise. Nevertheless, researchers at the University of California, Irvine, sought to enhance night vision by making an AI that fills in the blind spots and colorizes the images. For now, the colorization only works with portraits but in the not-so-distant future, it may be possible to colorize other features for a more natural feel that turns night into day.
The very first night vision equipment was made in the 1930s in Germany and saw use in World War II, but the US military wasn’t far behind and soon developed their own night vision tools called “sniperscopes”. The working principle of these “Generation 0” devices hasn’t changed in modern night vision goggles, although new devices are much smaller and more portable compared to their cumbersome early counterparts that required an operator to carry around a backpack-sized power source. Then and now, night vision devices featured an infra-red illuminator that hit and reflected off objects and surfaces, before bouncing back to the lens of the scope where a visible image was reconstructed by amplifying the tiny amounts of light in the infrared (non-visible) spectrum.
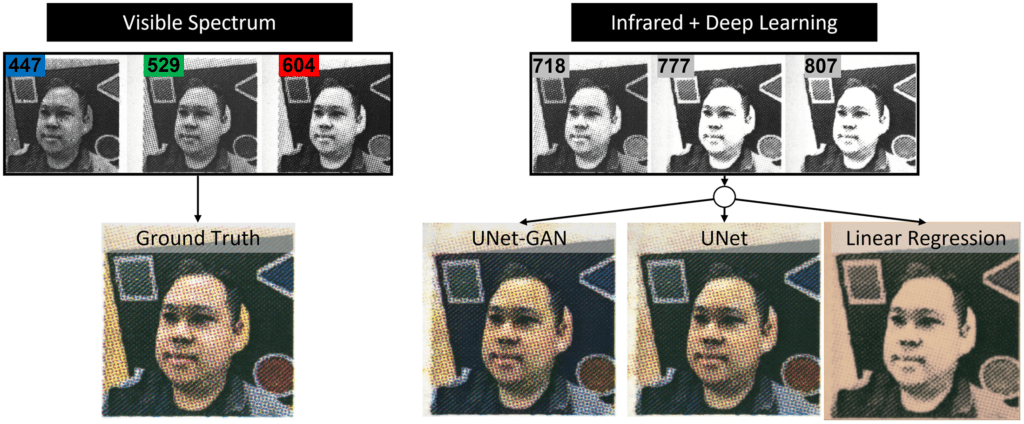
The images produced by night vision devices are all grayscale but for a number of reasons, a screen coated with phosphor is used to produce a characteristic green tint. Without going into too many technicalities, engineers found that the monochrome night vision image is most accurately perceived and distinguished in shades of green. That’s perhaps because the human eye is most sensitive to light wavelengths close to 555 nanometers, corresponding to the color green, allowing the display to be a little dimmer than for other colors, which helps a lot to conserve battery power.
While perfectly usable in its current configuration, researchers led by Andrew Browne from the University of California, Irvine, wanted to give night vision a touch of color. To this aim, they used a monochromatic camera sensitive to visible and near-infrared light to acquire images of printed photos showing 140 portraits. The researchers then optimized a type of AI called a convolutional neural network to predict what near-infrared images should look like in the visible spectrum.
“This study serves as a first step towards predicting human visible spectrum scenes from imperceptible near-infrared illumination. Further work can profoundly contribute to a variety of applications including night vision and studies of biological samples sensitive to visible light,” the researchers wrote in the journal PLOS ONE.
Although the produced visible spectrum images are impressive and almost indistinguishable from genuine color images, this system is limited to human faces. It can’t color night vision images showing vegetation, cityscapes, or vehicles. And since the AI works by spotting correlations between what little visible light and infrared is reflected by objects, the added colors are a form of educated guesses rather than an accurate portrayal of reality.

Colorizing a general scene captured by night vision devices may never be truly possible. Nevertheless, these findings are a promising step in that direction.
“This proof-of-principle study using printed images with a limited optical pigment context supports the notion that the deep learning approach could be refined and deployed in more practical scenarios. These scenarios include vision applications where little visible light is present either by necessity or by goal. Night vision, for example, has applications in security, military operations, and animal observation. Similarly, handling, processing, and studying samples sensitive to visible light may require a technology that uses no visible light,” the researchers concluded.