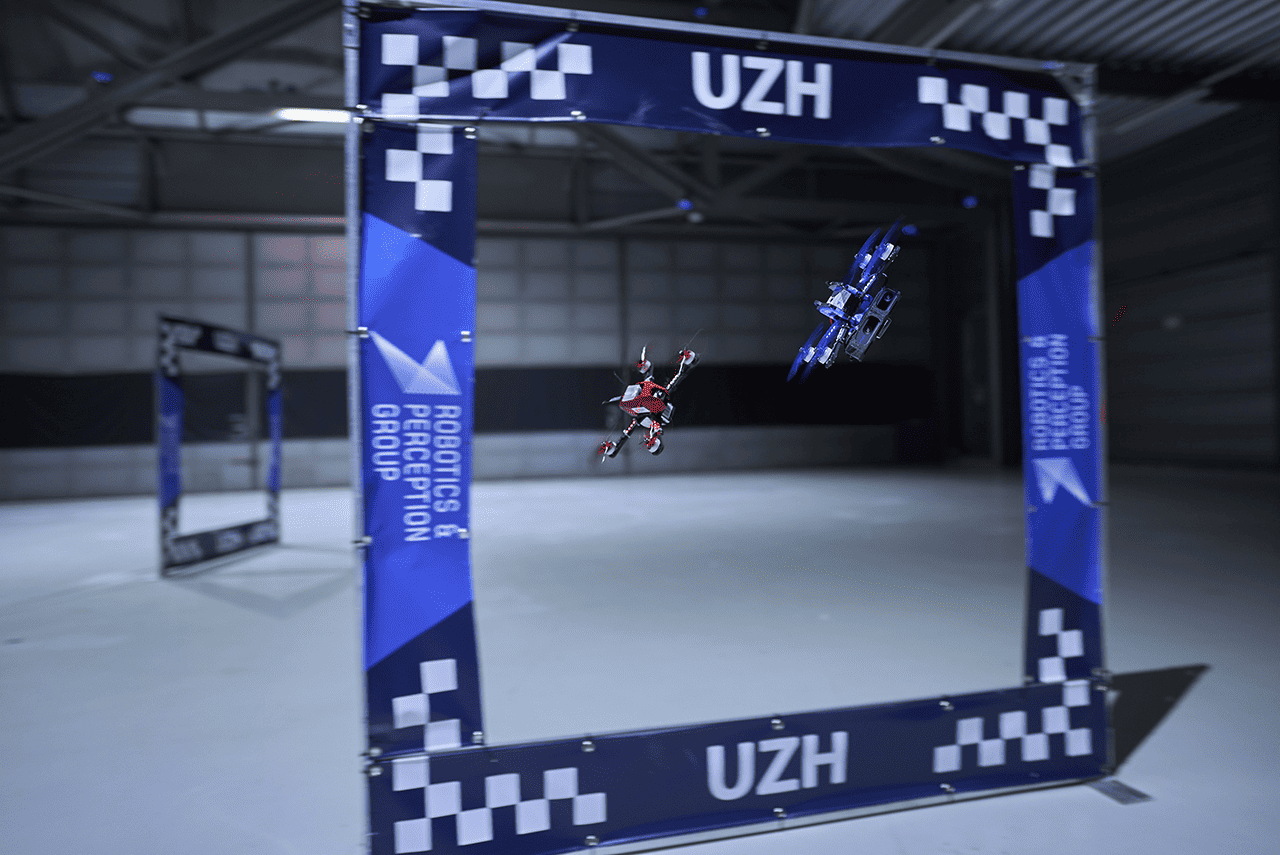
Artificial intelligence (AI) has outperformed even the best human players in various games and competitions. From chess to Go and even video games like Gran Turismo and DOTA 2, AI has demonstrated its prowess. Now, researchers at the University of Zurich have taken things to the next level with a remarkable autonomous drone system named “Swift.”
This AI-powered drone successfully outpaced human drone-racing champions, marking a significant milestone in the integration of AI with real-world activities.
Swift: The Autonomous Drone Champion
Swift, the autonomous drone developed by Elia Kaufmann and colleagues at the University of Zurich, beat three skilled human drone-racing champions in a real-world racing competition. Unlike purely digital environments where previous AI triumphs have occurred, this milestone is much more impressive because first-person view (FPV) drone racing is one of the most challenging types of racing in the world.
During FPV drone racing, pilots have to make very sharp turns at speeds exceeding 150 kilometers per hour through complex, obstacle-laden courses and make snap decisions in the blink of a moment. What sets this sport apart is the immersive experience it provides to the pilots.
Equipped with special goggles that stream live video footage from a camera mounted on the drone, pilots essentially see through the eyes of the drone, allowing them to navigate the course from a first-person perspective.
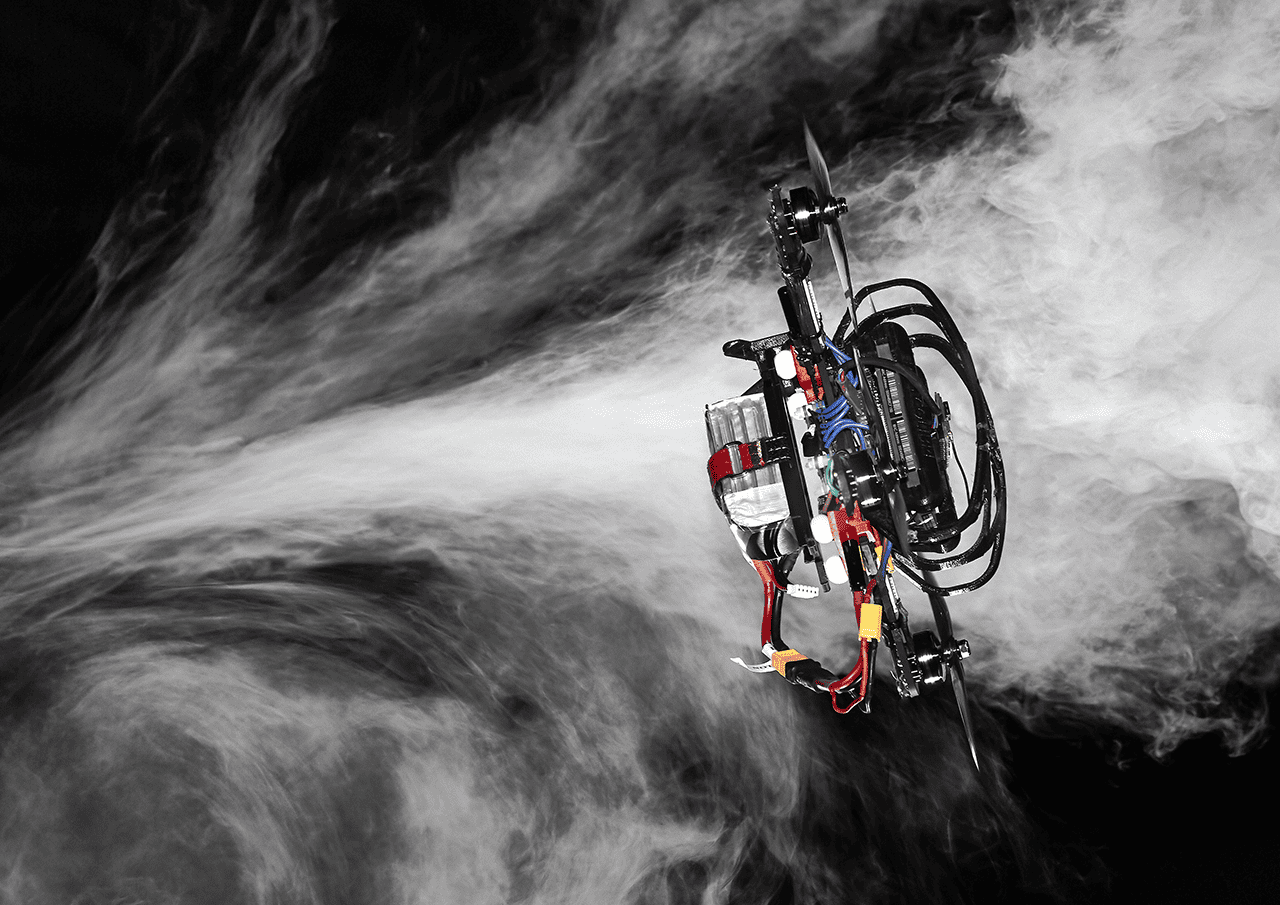
Unlike digital or tabletop games with limited rules, albeit with a high number of possible moves, an AI taking on drone racing needs to integrate motion-capture sensors and navigate a real-life environment in real-time that can be challenging to pull off — especially when you’re put head-to-head with amazing champions, such as Alex Vanover (2019 Drone Racing League champion), Marvin Schäpper (2021 Swiss Drone League champion), and Thomas Bitmatta (World Drone Cup 2019 champion).
Swift won 15 out of 25 races against each human champion. It also achieved the fastest recorded race time over the course at the University of Zurich, beating the best time clocked by a human pilot by half a second.
The success of Swift can be attributed to a combination of clever engineering and an innovative AI approach that relies on learning through trial and error. The standard practice for training AI robots involves end-to-end learning, wherein AI learns to map images to specific commands controlling the drone’s movements.
However, this approach often falls short when transitioning from simulated environments to real-world settings. In the field, this is often known as the “reality gap.”
Overcoming the Reality Gap

Swift’s approach was different. The team combined deep reinforcement learning — a technique that optimizes performance through trial and error — in simulation with data collected in the physical world. The process began with processing images captured by the drone’s camera, employing an artificial neural network to identify gate corners — a task at which AI excels.
The drone’s speed was determined using proprietary software from its binocular vision system. These two sets of information were then integrated with data from the inertial measurement unit, which measures acceleration and rotation rate, using conventional algorithms.
Swift’s innovative feature lies in its use of another artificial neural network that maps the drone’s state to commands to adjust its thrust and rotation. This second AI is used in a specific part of the process, known as abstraction, allowing the scientists to bridge the reality gap. This higher-level abstraction helped reduce the differences between simulations and real systems, enabling faster and more accurate learning.
The implications of Swift’s victory extend beyond the realm of drone racing. The technology could certainly have military applications, as the war in Ukraine has shown that drones are the future of warfare. But the broader range of potential uses could be even more exciting. Imagine drones that can safely navigate complex urban environments and perform all sorts of tasks ranging from cleaning windows on high-rise buildings to delivering food directly to your 10th-floor apartment.
Yet it may take a while before we see such developments. While Swift’s success is noteworthy, there are many challenges that remain. The tests were conducted in controlled indoor environments, while real drone races occur in more unpredictable settings with changing conditions, including wind, weather, and even other drones.
The findings appeared in the journal Nature.