Researchers at the University of Warwick have identified a host of new exoplanets from old NASA data with the use of machine learning.
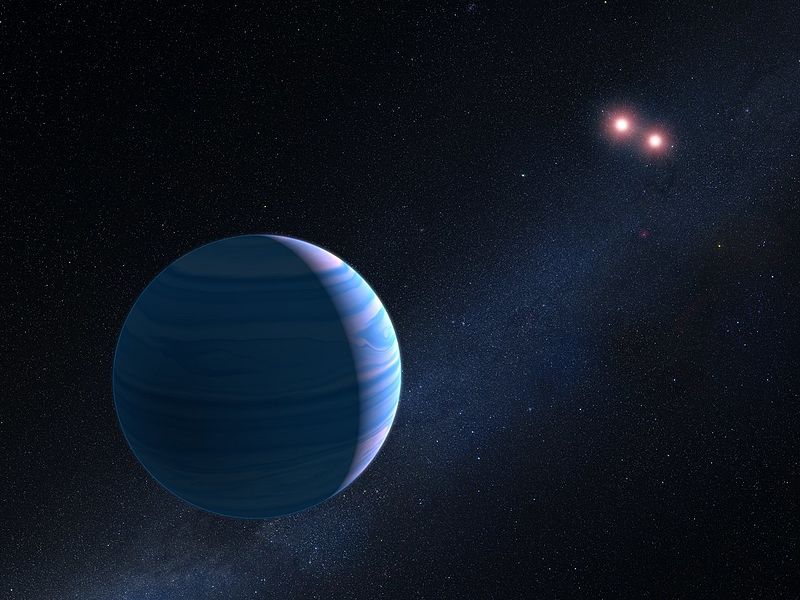
Image credits NASA, ESA, and G. Bacon (STScI).
Identifying planets far away from our own isn’t easy. It involves a painstaking process of waiting for the planet to come between its star and our telescope, which will temporarily block or reduce its brightness. Based on how much of the light is obscured, we can tell whether they’re caused by a planet or something else in the huge expanse of space. This is called the transit method.
We’re far from getting this process down to a T, simply because human beings aren’t very good at processing massive amounts of data at the same time. But machine learning is.
Planet App
“In terms of planet validation, no-one has used a machine learning technique before,” said David Armstrong of the University of Warwick, one of the study’s lead authors, in a news release.
“Machine learning has been used for ranking planetary candidates but never in a probabilistic framework, which is what you need to truly validate a planet.”
The team trained their algorithm using data from the Kepler Space Telescope, retired in 2018 after a nine-year mission. From this wealth of data, it learned to identify planets and to weed out false positives using feedback from the researchers. After it was trained, the team fed it older data sets, and the program found 50 exoplanets ranging from Neptune-sized gas giants to rocky worlds smaller than Earth. Their orbits (how long it takes them to go around their stars) range from around 200 days to some as short as a single day.
The team notes that smaller planets are particularly hard to spot with the transit method, so finding such planets showcases the ability of the AI. The next step is to take our existing tools and give these 50 planets a more thorough look-over.
Such an AI however will surely be used again. The ability to monitor huge areas of the night’s sky quickly and reliably could speed up our efforts to identify planets by a huge degree. It’s likely far from perfect now, but algorithms can be improved as our knowledge improves, the team notes.
“We still have to spend time training the algorithm, but once that is done it becomes much easier to apply it to future candidates,” Armstrong said.
The paper “Exoplanet Validation with Machine Learning: 50 new validated Kepler planets” has been published in the journal Monthly Notices of the Royal Astronomical Society.