Bad wells tend to be excluded from studies on groundwater quality which leads to biased assessments, a new study concludes. If this is the case, the state of the groundwater may worse than we thought in many parts of the world.
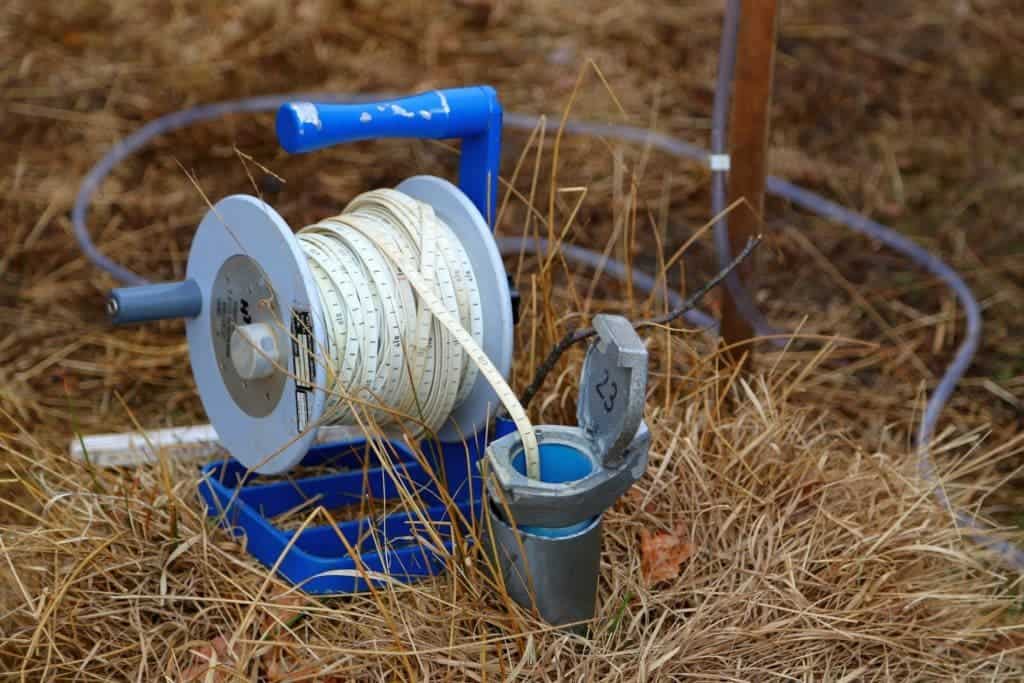
A fluid debate
Researchers from the University of Waterloo realized something was wrong with groundwater study when they noticed a major discrepancy between official data and anecdotal stories from southern India. According to data based on thousands of wells and satellite imagery, groundwater levels were rising steadily — which, in an area greatly relying on agriculture, is excellent. However, stories from farmers in the field told a different story.
Fieldworkers were hearing more and more stories from farmers about wells running dry — which suggests that levels were actually declining. It wasn’t just farmers complaining, there were also numerous reports of farmers digging deeper, more expensive wells because they couldn’t find water otherwise. The discrepancy was downright weird.
“If indeed groundwater levels are going up, why would farmers choose to pay more and dig deeper wells?” asked Nandita Basu, a civil and environmental engineering professor. “It didn’t make sense.”
In order to solve this issue, they combed through previous studies on groundwater, finding that wells with missing water levels were often excluded from the analysis because they were considered unreliable as data points. When these wells were added back to the data, the resulting picture fit much better with the stories from local farmers.
“They were systematically picking the wells with a lot of data and potentially ignoring the wells that were going dry because they had incomplete data,” said Tejasvi Hora, an engineering Ph.D. student who led the research.
A global issue
While the study was carried out on data from India, similar things are probably happening in many parts of the world.
It’s not just groundwater studies, either. Survivor bias artifacts can be easy to miss in science, producing numerous misleading results if not handled carefully. A quite literal example comes from a 1987 study on cats, which found that cats falling from more than six stories up are likely to have fewer injuries than cats falling from under six stories high — something which is probably caused by the fact that cats falling from more than six stories are often killed, and don’t end up in the vet for an injury report.
Similar examples of survivor bias in science appear in numerous fields of science, and researchers call more attention when it comes to data selection.
“Our main point is that bad data is good data,” she said. “When you have wells with a lot of missing data points, that is telling you something important. Take notice of it.”
“Whenever you’re focusing only on complete data, you should take a step back and ask if there is a reason for the incomplete data, a systematic bias in your data source,” Hora said.
The study was published in Geophysical Research Letters.