Every time you speak, your neck and facial muscles move in a specific way. Many people with speech impediments are still able to move their muscles, despite not being able to talk smoothly. Now, researchers are looking at a new way to use technology to reverse engineer these muscle movements and translate them into a synthetic audible voice.
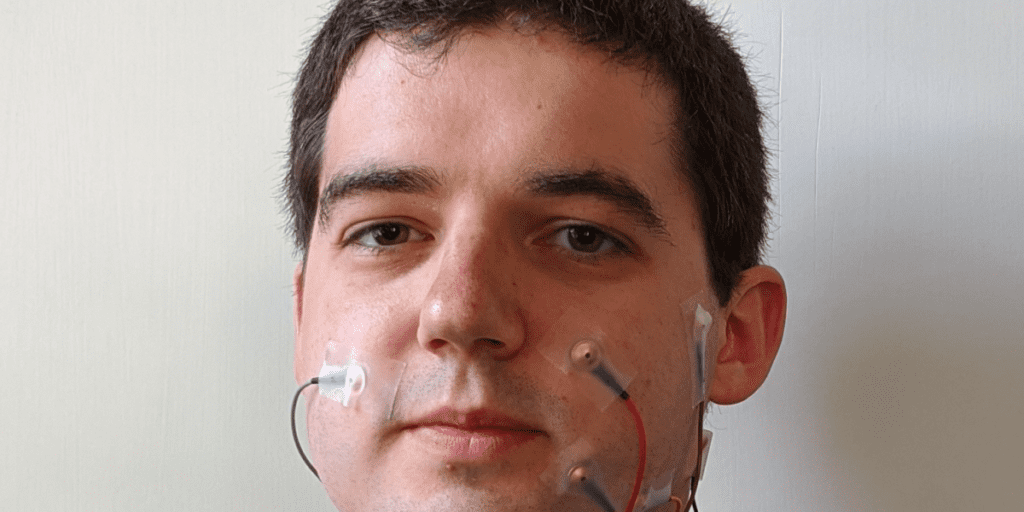
The approach developed by UC Berkeley researchers uses electrodes placed on the face and throat. Broadly speaking, the method is called electromyography (or EMG) — where electrode sensors collect information about muscle activity. An algorithm then builds a model of the muscle data and generates synthetic speech. It’s a sort of electronic lip reading, except than it doesn’t use the actual lip movements for tracking facial movements.
“Digitally voicing silent speech has a wide array of potential applications,” the team’s paper reads. “For example, it could be used to create a device analogous to a Bluetooth headset that allows people to carry on phone conversations without disrupting those around them. Such a device could also be useful in settings where the environment is too loud to capture audible speech or where maintaining silence is important.”
It’s not the first time something like this has been developed. Silent speech interfaces have been around for a few years, but there’s still plenty of room for improvement when it comes to the performance of these devices. This is where the new approach comes in with an innovation: the AI algorithm transfers audio outputs “from vocalized recordings to silent recordings of the same utterances.” In other words, this is the first model that trains the algorithm with EMG data collected during silent speech, not ‘real’ speech. This approach offers better performance, the researchers note in the study.
“Our method greatly improves intelligibility of audio generated from silent EMG compared to a baseline that only trains with vocalized data,” the researchers add.
According to the measured data, the word interpretations produced this way were more accurate than existing technology. In one experiment, transcription word error dropped from 64% to 4%, while in another experiment (which used a different vocabulary), it dropped from 88% to 68%.
The paper has been published in the journal arXiv and has not yet been peer reviewed at the time of this writing. However, the paper has received an award at the Empirical Methods in Natural Language Processing (EMNLP) event held online last week, in recognition of its results.
To support more research in this field, researchers have open-sourced a dataset of nearly 20 hours of facial EMG data.