Researchers have created a working artificial, organic synapse. The new device could allow computers to mimic some of the brain’s inner workings and improve their capacity to learn. Furthermore, a machine based on these synapses would be much more energy efficient that modern computers.
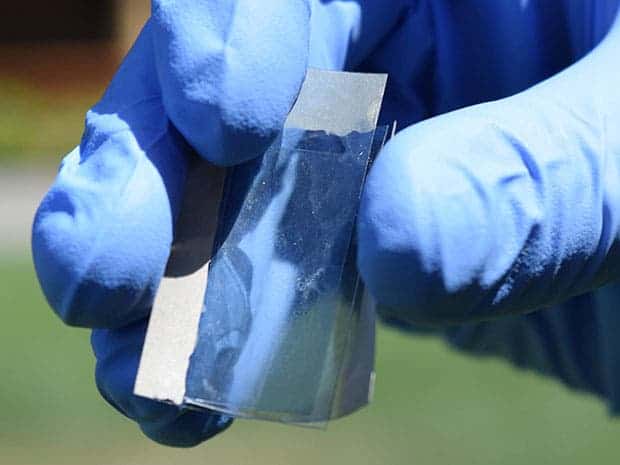
Image credits Stanford University.
As far as processors go, the human brain is hands down the best we’ve ever seen. Its sheer processing power dwarfs anything humans have put together, for a fraction of the energy consumption, and it does it with elegance. If you allow me a car analogy, the human brain is a formula 1 race car that somehow uses almost no fuel and our best supercomputer… Well, it’s an old, beat-down Moskvich.
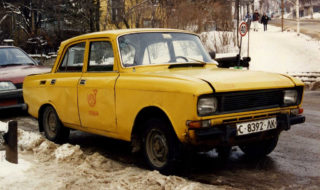
Image credits Sludge G / Flickr.
So finding a way to emulate the brain’s hardware has understandably been high on the wishlist of computer engineers. A wish that may be granted sooner than they hoped. Researchers Stanford University and Sandia National Laboratories have made a breakthrough that could allow computers to mimic one element of the brain — the synapse.
“It works like a real synapse but it’s an organic electronic device that can be engineered,” said Alberto Salleo, associate professor of materials science and engineering at Stanford and senior author of the paper.
“It’s an entirely new family of devices because this type of architecture has not been shown before. For many key metrics, it also performs better than anything that’s been done before with inorganics.”
Copycat
The artificial synapse is made up of two thin, flexible films holding three embedded terminals connected by salty water. It works similarly to a transistor, with one of the terminals dictating how much electricity can flow between the other two. This behavior allowed the team to mimic the processes that go on inside the brain — as they zap information from one another, neurons create ‘pathways’ of sorts through which electrical impulses can travel faster. Every successful impulse requires less energy to pass through the synapse. For the most part, we believe that these pathways allow synapses to store information while they process it for comparatively little energy expenditure.
Because the artificial synapse mimics the way synapses in the brain respond to signals, it removes the need to separately store information after processing — just like in our brains, the processing creates the memory. These two tasks are fulfilled simultaneously for less energy than other versions of brain-like computing. The synapse could allow for a much more energy-efficient class of computers to be created, addressing a problem that’s becoming more and more poignant in today’s world.
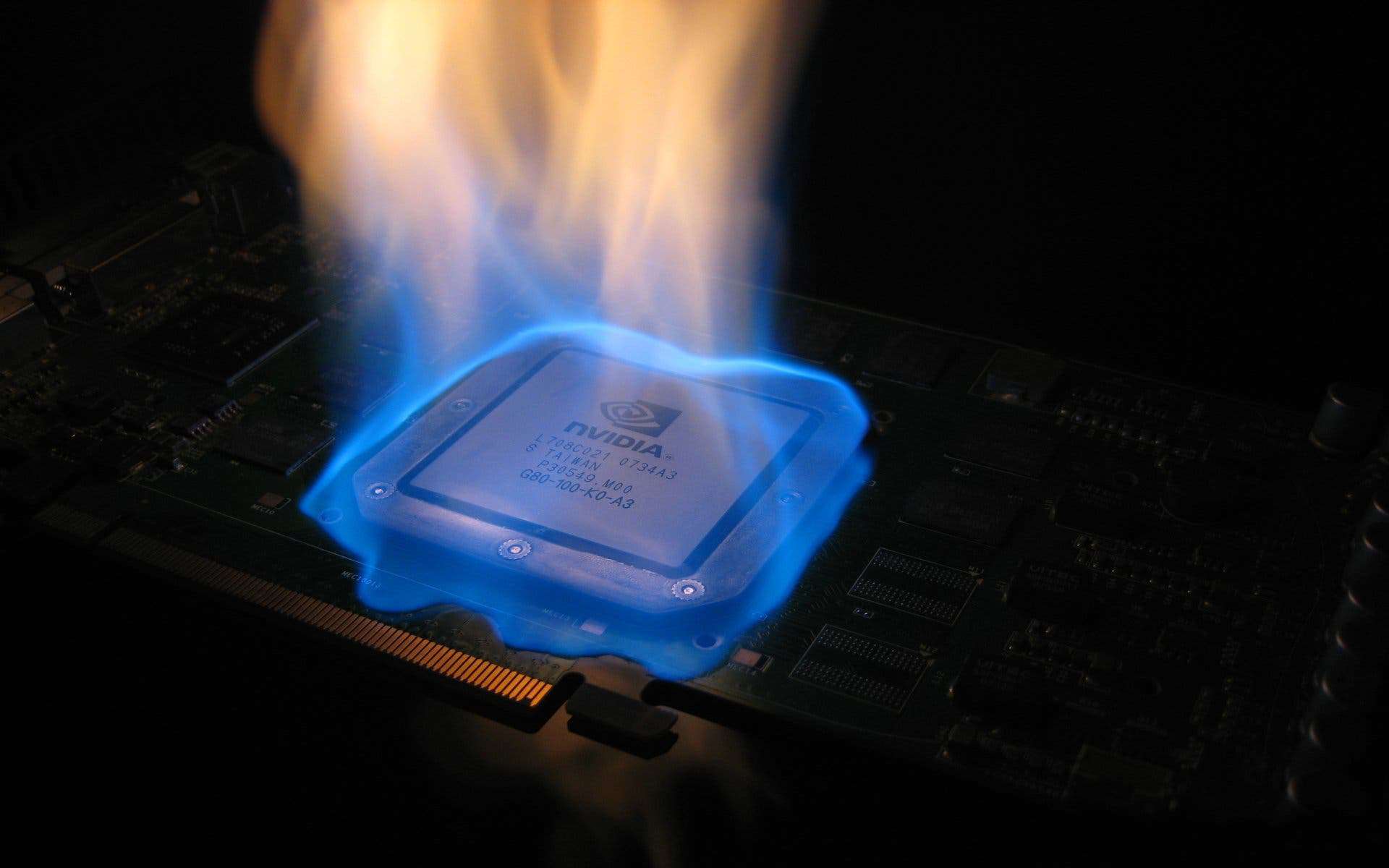
One application for the team’s synapses could be more brain-like computers that are especially well suited to tasks that involve visual or auditory signals — voice-controlled interfaces or driverless cars, for example. Previous neural networks and artificially intelligent algorithms used for these tasks are impressive but come nowhere near the processing power our brains hold in their tiny synapses. They also use a lot more energy.
“Deep learning algorithms are very powerful but they rely on processors to calculate and simulate the electrical states and store them somewhere else, which is inefficient in terms of energy and time,” said Yoeri van de Burgt, former postdoctoral scholar in the Salleo lab and lead author of the paper.
“Instead of simulating a neural network, our work is trying to make a neural network.”
The team will program these artificial synapses the same way our brain learns — they will progressively reinforce the pathways through repeated charge and discharge. They found that this method allows them to predict what voltage will be required to get a synapse to a specific electrical state and hold it with only 1% uncertainty. Unlike traditional hard drives where data has to be stored or lost when the machine shuts down, the neural network can just pick up where it left off without the need for any data banks.
One of a kind
Right now, the team has only produced one such synapse. Sandia researchers have taken some 15,000 measurements during various tests of the device to simulate the activity of a whole array of them. This simulated network was able to identify handwritten digits (between 0-9) with 93 to 97% accuracy — which, if you’ve ever used the recognize handwriting feature, you’ll recognize as an incredible success rate.
“More and more, the kinds of tasks that we expect our computing devices to do require computing that mimics the brain because using traditional computing to perform these tasks is becoming really power hungry,” said A. Alec Talin, distinguished member of technical staff at Sandia National Laboratories in Livermore, California, and senior author of the paper.
“We’ve demonstrated a device that’s ideal for running these type of algorithms and that consumes a lot less power.”
One of the reasons these synapses perform so well is the numbers of states they can hold. Digital transistors (such as the ones in your computer/smartphone) are binary — they can either be in state 1 or 0. The team has been able to successfully program 500 states in the synapse, and the higher the number the more powerful a neural network computational model becomes. Switching from one state to another required roughly a tenth of the energy modern computing system drain to move data from processors to memory storage.
Still, this means that the artificial synapse is currently 10,000 times less energy efficient than its biological counterpart. The team hopes they can tweak and improve the device after trials in working devices to bring this energy requirement down.
Another exciting possibility is the use of these synapses in-vivo. The devices are largely composed of organic elements such as hydrogen or carbon, and should be fully compatible with the brain’s chemistry. They’re soft and flexible, and use the same voltages as those of human neurons. All this raises the possibility of using the artificial synapse in concert with live neurons in improved brain-machine interfaces.
Before they considering any biological applications, however, the team wants to test a full array of artificial synapses.
The full paper “A non-volatile organic electrochemical device as a low-voltage artificial synapse for neuromorphic computing” has been published in the journal Nature Materials.