A new artificial intelligence system can translate a person’s brain activity, while listening to a story or imagining telling a story, into a continuous stream of text. The system, called a semantic decoder, might one day help people who are mentally conscious but unable to physically speak to communicate intelligibly once again.
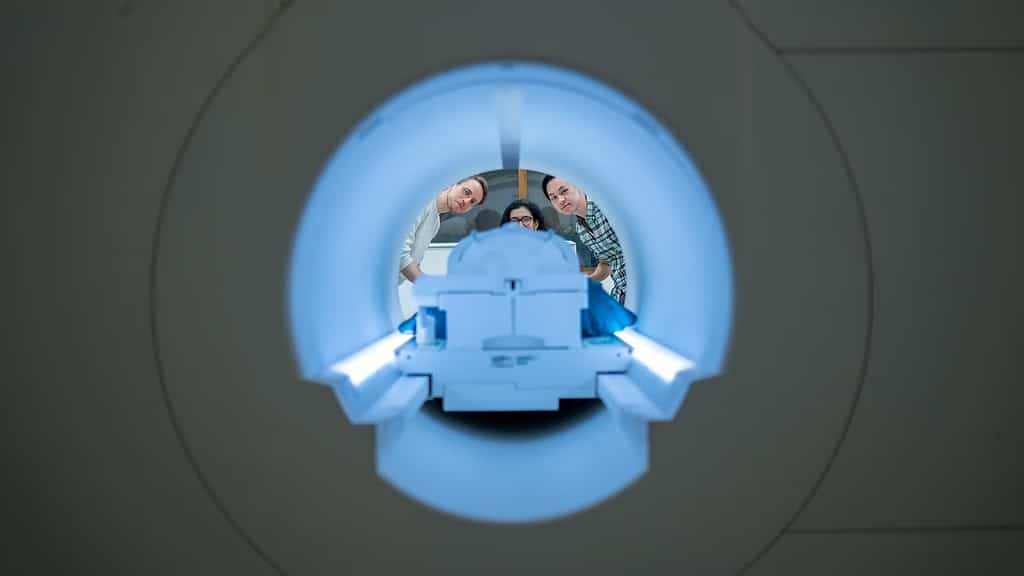
The system relies in part on a transformer model, similar to the ones that power Google’s Bard and Open AI’s ChatGPT. Unlike other language decoding systems in the works, the system doesn’t require people to have surgical implants – making it noninvasive. Participants also don’t need to only use words from a prescribed list.
Instead, brain activity is measured using an fMRI scanner after extensive training of the decoder, in which the individual listens to podcasts in the scanner. Later, if the subject is open to having their thoughts decoded, their listening to a new story or imagining telling a story allows the machine to generate text from brain activity alone.
The result is not a transcript. The researchers created it to capture the main ideas of what is being said or thought by the subject, although imperfectly. Half the time, when the system was trained to monitor a participant’s brain activity, the machine created text that closely and precisely matches the intended meaning of the original words.
“For a noninvasive method, this is a real leap forward compared to what’s been done before, which is typically single words or short sentences,” Alex Huth, a neuroscience researcher at the University of Texas at Austin and study author, said in a statement. “We’re getting the model to decode continuous language for extended periods of time with complicated ideas.”
A big breakthrough
The study overcomes a limitation of fMRI. While it can map brain activity to a specific location with high resolution, there’s a time lag – which makes real-time tracking impossible. The lag happens because scans detect blood flow response to brain activity, which reaches its peak and then returns to baseline levels in about 10 seconds.
This limitation has hindered the understanding of brain activity during natural speech. However, language models such as ChatGPT can convert speech into semantic meaning, enabling researchers to observe brain activity that corresponds to strings of words with a specific significance, rather than analyzing activity word-by-word.
For the study, the researchers asked three volunteers to lie in a scanner for 16 hours and listen to narrative podcasts such as The Moth. The system was trained to match brain activity to meaning. The volunteers were then scanned listening to a new story or imagining telling a story and the system had to generate text just using brain activity.
For example, when a participant heard a speaker say, “I don’t have my driver’s license yet,” their thoughts were interpreted as, “She has not yet begun to learn how to drive.” Similarly, upon listening to the words, “I didn’t know whether to scream, cry or run away. Instead, I said, ‘Leave me alone!’,” the participant’s neural activity was decoded as, “She began to scream and cry, but eventually instructed the speaker to leave her alone.”
At present, the system’s dependence on fMRI machine makes it unsuitable for usage beyond laboratory settings. However, the researchers believe it could be applied to other brain imaging systems, such as functional near-infrared spectroscopy (fNIRS), which are more portable. “Our exact kind of approach should translate to fNIRS,” Huth said in a statement.
The study was published in the journal Nature Neuroscience.