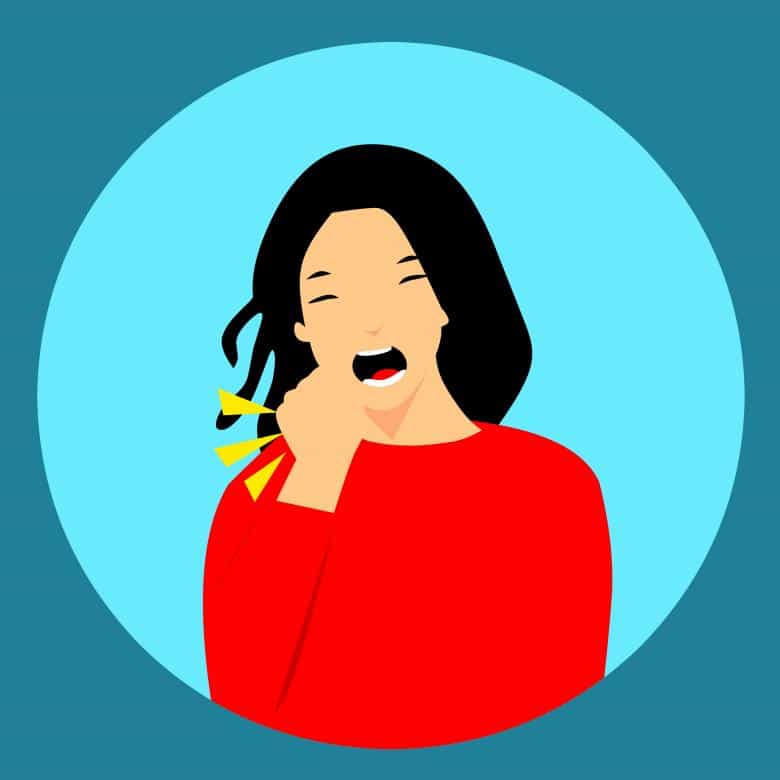
Pneumonia, an infection that inflames the air sacs in one or both lungs, accounts for around 14% of all deaths in children under 5 years old, killing over 700,000 children a year. Most adults recover from pneumonia, especially with treatment, but for children and those with less potent immune systems, it can be a devastating disease — especially in the less developed parts of the world, where treatment options may be scarce.
Needless to say, diagnosing pneumonia early, quickly, and cheaply could make a big difference. Currently, diagnosing pneumonia is usually done through blood tests and chest scans, but these can be expensive, and time-consuming, and a doctor needs to suspect pneumonia to request them. But there could be a tell-tale sign of pneumonia that can be used to diagnose the disease without any of those: coughing.
Cough into this algorithm
Pneumonia coughs aren’t like other coughs — because the disease comes with an inflammation of the lungs that modifies the airwave coming from a sound. For humans, it’s hard to pick up on the difference. But with the help of specialized electronic equipment, and an algorithm to oversee the process, it can be done.
At the 183rd Meeting of the Acoustical Society of America, Jin Yong Jeon of Hanyang University presented a machine learning algorithm that does just that: it identifies whether a patient has pneumonia by sound analysis of a cough. The machine learning algorithm is first trained to differentiate coughs from pneumonia from coughs from non-pneumonia sources, and then, once it becomes competent enough, it is used for diagnosis.
This isn’t the first time something like this was developed. Several other research teams have been working on diagnosing pneumonia or monitoring lung health, sometimes with a simple smartphone microphone. By mixing cheap sensors and smart algorithms, researchers want to create new ways to diagnose diseases cheaply and effectively.

In this case, Jeon and colleagues augmented the recordings with room impulse responses, which measure how the acoustics of a space influences different sound frequencies. By combining this with the recorded cough sounds, the algorithm can work more efficiently in any environment. Overall, the researchers were able to achieve 97.5% accuracy for the dataset.
“Automatically diagnosing a health condition through information on coughing sounds that occur continuously during daily life will facilitate non-face-to-face treatment,” said Jeon. “It will also be possible to reduce overall medical costs.”
Already, one company has expressed plans to use the algorithm for remote patient monitoring. If it could be made to work with simple equipment, in any environment, it could make a huge difference for the millions of vulnerable patients who get pneumonia every year.
“Our research team is planning to automate each step-by-step process that is currently performed manually to improve convenience and applicability,” said Jeon.
Diagnosing pneumonia is far from an isolated case. AI is increasingly playing a more prominent role in diagnosing diseases. From heart disease and Parkinson’s to childhood diseases, algorithms are showing promise to aid doctors in making a diagnosis — because ultimately, the goal isn’t to replace medical practitioners, but rather to complement their work and make it easier for them to come to a conclusion.
This type of technology could be impactful across different diseases to aid early diagnosis and intervention.
For now, however, the paper was presented at a conference and has not been peer-reviewed yet.