Historical maps are an important tool for analyzing changes in urban development. But these maps only provide 2D information, and oftentimes not much is known about building height.
With this in mind, a research team from Skoltech (Russia) and FBK (Italy) developed a machine learning algorithm that can assess building height from historical maps, which could help researchers better understand the forces that shaped cities to what we see today.

Nowadays, we’ve become pretty used to having 3D maps at our disposal, but this is a luxury we take for granted too easily. For the vast majority of our history, maps and urban imagery were restricted to 2D information.
A team of researchers led by Elisa Mariarosaria Farella from the Fondazione Bruno Kessler (FBK) in Trento, Italy, wanted to see how the height of buildings in historical maps can be inferred. They developed a machine learning algorithm that was first trained on sample data, and then asked it to predict buildings’ heights.
The algorithm’s assessment is based on several “predictors” — variables that are assumed to have some connection (not necessarily a direct or causal connection) to the objective (in this case, the building height). Researchers used things like a building area, its type (whether it was residential, historical, etc), its distance to other buildings, and so on.
They then ground-truthed the model predictions and finessed it with historical photos (where available) and improved mathematical algorithms, using four historical maps of Trento (years 1851, 1887, 1908, and 1936) and Bologna (years 1884 and 1945),
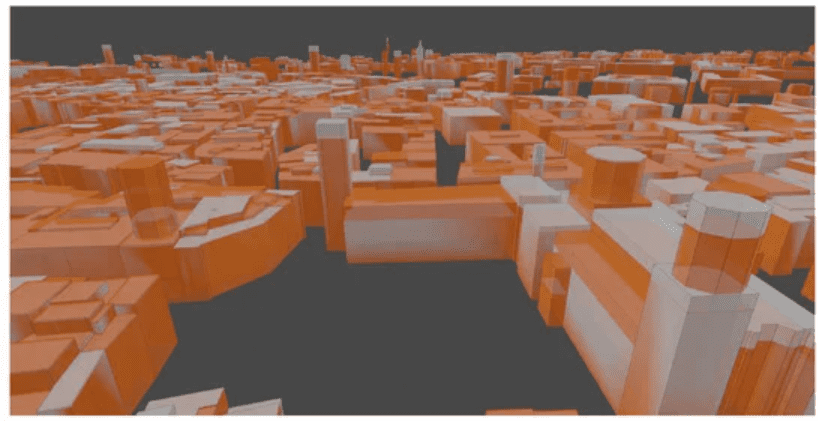
Overall, the results were good, researchers write in the study.
“The reliability of the proposed approach was verified, testing the method on different datasets and epochs. The achieved results proved to be consistent with our accuracy targets and the complexity of such historical urban contexts. The implemented method is flexible and extendable, relying mainly on geometric and neighbour characteristics derivable from the datasets and adaptable categorical data.”
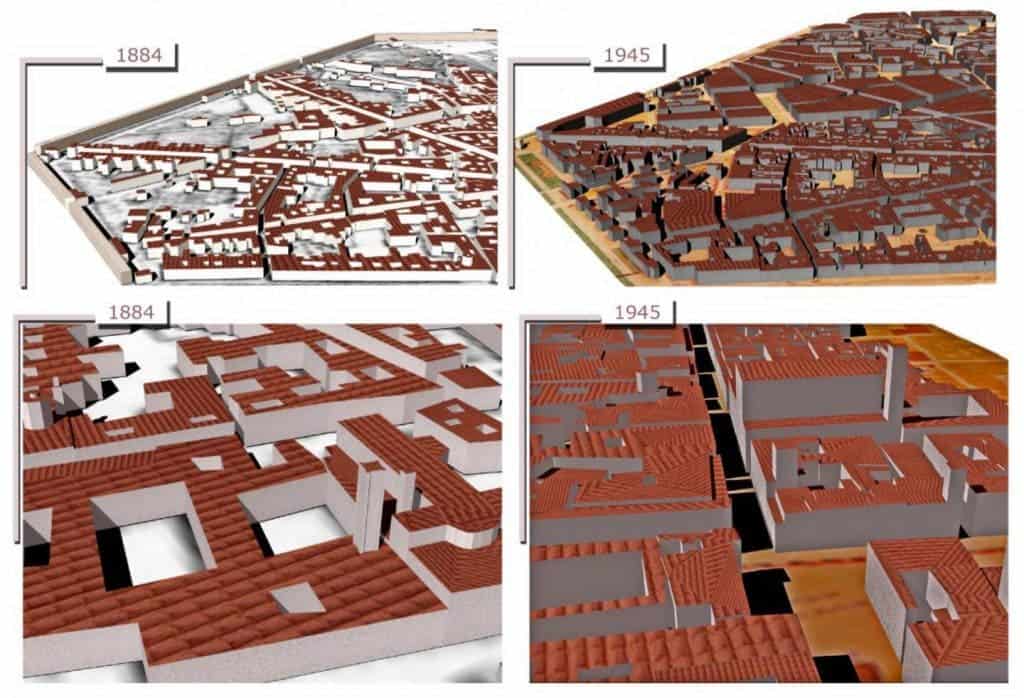
They are now looking at ways to improve the process even further and generalize the method, which could make it much easier to digitize historical maps from all around the world and enhance them to 3D.
“The implemented learning and predictive procedure tested on historical data has proved to be effective and promising for many other applications. Based on few attributes for the prediction, it will soon be expanded to diverse real-life contexts with missing elevation data. The resulting models will be a great help in bridging the geospatial knowledge gap in past or remote situations” Emre Ozdemir, a Skoltech and FBK Trento PhD student, explains.
The study was published in MDPI Applied Sciences.