The algorithm is surprisingly good at spotting changes associated with Alzheimer’s disease, giving patients crucial time to intervene with treatment.
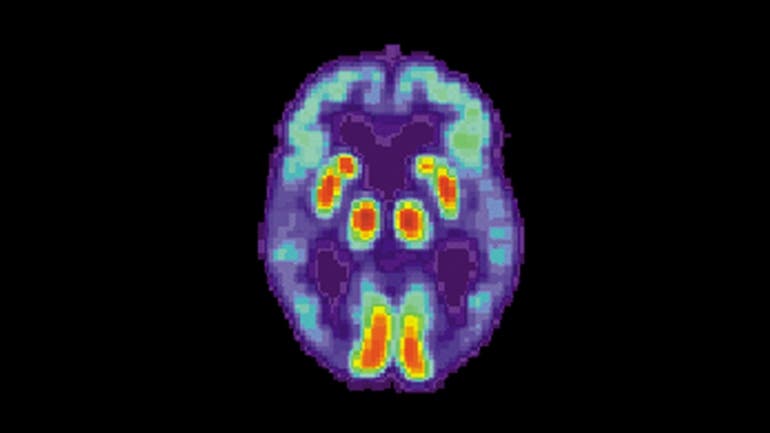
As with many other conditions, early detection is crucial in Alzheimer’s. The problem, however, is that the symptoms aren’t usually clear — and by the time a diagnosis is done, most treatments lose efficacy. Although there is no direct treatment for Alzheimer’s, there are several treatments that can help stem the progress of the condition. Having a way to diagnose it sooner could make a huge difference in dealing with the disease.
“One of the difficulties with Alzheimer’s disease is that by the time all the clinical symptoms manifest and we can make a definitive diagnosis, too many neurons have died, making it essentially irreversible,” says Jae Ho Sohn, MD, MS, a resident in the Department of Radiology and Biomedical Imaging at UC San Francisco.
This is where the new AI comes in. Sohn and colleagues combined neuroimaging with machine learning, “training” the algorithm to analyze PET scans and look for Alzheimer’s-related patterns. They first fed it images from the Alzheimer’s Disease Neuroimaging Initiative (ADNI), a massive public dataset of PET scans from patients who were eventually diagnosed with either Alzheimer’s disease, mild cognitive impairment or no disorder.

“This is an ideal application of deep learning because it is particularly strong at finding very subtle but diffuse processes. Human radiologists are really strong at identifying tiny focal finding like a brain tumor, but we struggle at detecting more slow, global changes,” says Sohn. “Given the strength of deep learning in this type of application, especially compared to humans, it seemed like a natural application.”
After 1,921 training scans, they fed it another 188 images — also from the same database, but not previously presented to the AI. An additional set of 40 scans from patients who had presented to the UCSF Memory and Aging Center with possible cognitive impairment. The AI passed with flying colors.
First of all, it was able to successfully identify 92% of patients who developed Alzheimer’s disease in the first test set and 98% in the second test set. Even more impressively, on average, it made the predictions 75.8 months (over 6 years) before the patient received the official diagnosis.
An algorithm of this type could be a very useful predictive tool for Alzheimer’s treatment. Of course, it will still be a while before the method can be clinically applied, but researchers are optimistic. Sohn wants to test the algorithm. But with additional validation and calibration in a larger setting, the device can be used, researchers say.
“I believe this algorithm has the strong potential to be clinically relevant,” Sohn concludes. The study has been published in Radiology.